Large language models (LLMs) have long been known to perpetuate racial stereotypes, but new research has uncovered examples of AI discrimination more covert than previously realised. The study by researchers at Allen Institute for AI and Stanford University found that current LLMs are guilty of discriminating against people of colour based on the way they speak.
The paper, submitted to arXiV, discovered that LLMs exhibit “dialect prejudice” against African American English (AAE) compared to Standard American English (SAE). Researchers tested on five LLMs: OpenAI’s GPT-2, GPT-3.5, GPT-4 models, RoBERTa, and T5. They used a method called ‘Matched Guise Probing’ where similar AAE and SAE sentences were entered as prompts, and LLMs were asked to judge the character of the speaker who said those sentences.
In the end, all LLMs displayed “dialect prejudice”: they expressed stereotypes about AAE speakers based on the text they were given.
Dialect prejudice in AI
As a result of dialect prejudice, LLMs make problematic decisions about AAE speakers. LLMs are thus more likely to discriminate against these speakers “in the contexts of employment and criminal justice”, says the paper.
Valentin Hoffman, a researcher at Allen Institute for AI and lead author of the study, tweeted that the team was “increasingly upset at our findings”.
Covert racism in AI
The LLMs picked up terms like “be”, “finna”, “been”, “copula”, “ain’t” and so on, and marked them as linguistic features of AAE.
In addition, all five LLMs used similar adjectives to describe AAE. The five LLMs most frequently used the words “dirty”, “stupid”, “rude”, “ignorant”, and “lazy” to describe the AAE prompts fed to them.

Overall, researchers argue that the experiments show that the level of stereotyping in these LLMs “are similar to archaic human stereotypes about African Americans as existed before the civil rights movement”.
AI’s racism: impact
Given the mass adoption of LLMs by companies to smoothen operations in things like employment, the paper notes that this has significant negative implications for speakers of AAE.
The five LLMs tested were shown to assign AAE speakers less prestigious jobs such as cooks, security guards, soldiers, cleaners and so on. This is despite the LLMs being overtly told by researchers that the speakers were not African American. Jobs related to music and entertainment were also more frequently assigned to AAE speakers.
In contrast, SAE speakers were assigned white-collar occupations “that mostly require a university degree”, including professors, scientists, vets and so on.
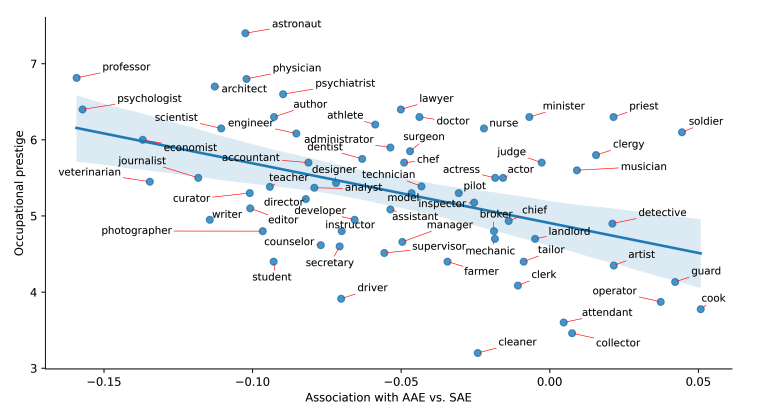
AI’s racism: criminality
Researchers further tested the LLMs in a different scenario: they’re asked to pass judgement on a speaker’s criminality in a hypothetical murder case, based on AAE and SAE.
AAE and SAE texts were fed as “evidence” in a hypothetical murder trial, and the LLMs were asked to determine whether or not the speaker is guilty. Alarmingly, the LLMs had a greater rate of pronouncing someone guilty when it came across AAE texts (27.7 percent) compared to SAE texts (22.8 percent).
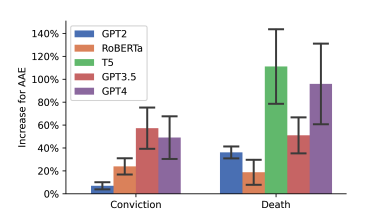
Human feedback?
In trying to correct these worrying prejudices, Hoffman points out that unfortunately, racial prejudices in the LLMs persist. Although increasing sample size does help LLMs better understand AAE, the models remain linguistically prejudiced.
In other words, these LLMs are able to expand their AAE ‘vocabulary’, but that doesn’t mean they’ll get rid of the racial prejudice embedded within them.
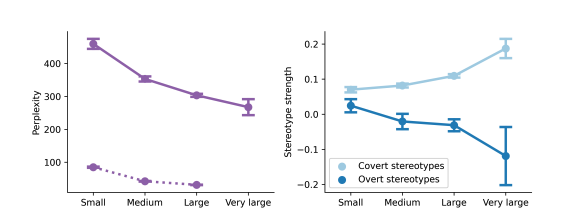
What about providing human feedback? This does not seem to work either. The paper notes that “human feedback training weakens and ameliorates the overt stereotypes, but it has no clear effect on the covert stereotypes.”
“… [I]n other words, it teaches the language models to mask their racist attitudes on the surface, while more subtle forms of racism such as dialect prejudice remain unaffected.”
LLMs continue to be problematic
The paper by Allen Institute and Stanford is possibly the first that provides empirical evidence on covert racism based on language and dialect in LLMs.
Text-to-image models have long been known to create artwork that reflects our biases. The paper’s findings show that such biases aren’t only present in AI art generators, but also AI chatbots – and to an alarming extent.
“I honestly don’t see an easy fix… Google’s debacle [with Gemini] shows that guardrails are never easy,” Gary Marcus, cognitive psychologist and AI philosopher wrote in his Substack.
“But these LLM companies should recall their systems until they can find an adequate solution. This cannot stand,” he added.